- August 14, 2020
- Posted by: Data Science Training and Solution
- Category: Data Analysis and Virtualization, Data Science Free Training
No Comments
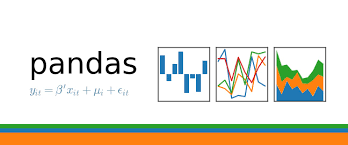
Numpy and Pandas
Numpy is a python package that is used for scientific computing. It provides support for large multi-dimensional arrays and matrices. Pandas is a python library used for data manipulation and analysis. Having a solid knowledge of both libraries is extremely useful for feature engineering, data imputation, and model building
>>> import numpy as np
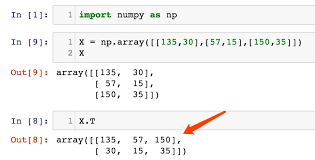
Some of the important attributes of a NumPy object are:
- Ndim: displays the dimension of the array
- Shape: returns a tuple of integers indicating the size of the array
- Size: returns the total number of elements in the NumPy array
- Dtype: returns the type of elements in the array, i.e., int64, character
- Itemsize: returns the size in bytes of each item
- Reshape: Reshapes the NumPy array
# list bob =[1,2,3] print(bob) import numpy as np # In[3]: # 1-Dimensional array tuna =[1,3,4,5,6,7,8] np.array(tuna) # In[4]: # 2-Dimensional array mat = ([1,2,3],[4,5,6],[5,6,7]) np.array(mat) # In[5]: #note that when dealing with 2-D # it always end with 2 ']]' of that # and if it is three of it ']]]' then it is 3-D # In[6]: np.arange(0,11) # In[7]: # when dealing with range in array we use arange np.arange(0,11,2) # the last parameter is the step(even num) # In[11]: # means 9 dot(.) np.zeros(9)+2 # In[10]: np.zeros((2,4)) # 2 -> num of rows # 4 -> num of columns # In[11]: np.ones((2,5)) # In[ ]: # In[12]: # linspace will take the number of POINT(dot. ) that we want e.g below is 5 np.linspace(0,5,5) # In[13]: #creating identity matrix # it a 2-D -> number of rows = number of colums np.eye(5, dtype=int) # In[14]: #the random library can also be used by from numpy.random import randint randint(2,7) #it give a random num from btw 2->6 # In[15]: # arrays of numbers = 1-D np.random.rand(5) # In[16]: np.random.rand(5,5) # 2-D # In[17]: np.random.randn(4,2) #return num < 0 # In[18]: # it give a random num btw the number but exclusive 100 np.random.randint(0,100) # In[19]: np.random.randint(56,100,10) #10 means 10 random numbers will be displayed butexclusive 100 # In[20]: #attribute of an array arr = np.arange(25) arr # In[21]: # reshape is used to return the data of an array # e.g arr above is 25 element and can be RESHAPED as 5x5 = 25 arr.reshape(5,5) # In[22]: rar = np.random.randint(0,51,10) rar # In[23]: rar.reshape(2,5) # In[93]: #to return the maximum and minimum of an array rar # In[94]: rar.max() # In[95]: rar.min() # In[96]: #getting the index location of the max value rar.argmax() # In[98]: #getting the index location of the min value rar.argmin() # In[99]: #getting the shape of a vector = size of array (1-D) rar.shape # In[29]: rar = rar.reshape(2,5) rar.shape # In[106]: #getting datatype arr.dtype # In[107]: rar.dtype # In[11]: np.full((5,4),3.14) # In[24]: mat = np.arange(1,26).reshape(5,5) mat # In[25]: # NB all counting start from 0 for both rows and cloumn mat[2:] # count row 2 down # In[26]: mat[2:,1:] # 1: reps column # In[28]: mat[3:,3:]
Learn and Earn More- PowerBI PL300 Certification: Your Guide to Advanced Data Visualization
Powered by Inline Related Posts
>>> import pandas as pd
Some commonly used data structures in pandas are:
- Series objects: 1D array, similar to a column in a spreadsheet
- DataFrame objects: 2D table, similar to a spreadsheet
- Panel objects: Dictionary of DataFrames, similar to sheet in MS Excel
# In[1]: import pandas as pd # In[2]: import numpy as np # In[20]: labs = ['a','b','c'] my_data = [11,30,40] arr = np.array(my_data) d = { 'a': 20, 'b':30,'c':40} # In[21]: pd.Series(data = my_data) # In[22]: pd.Series(data = my_data, index=labs) # In[23]: #OR pd.Series(my_data,labs) # In[24]: pd.Series(arr,d) # In[25]: pd.Series(d) # In[14]: # a pandas series can hold a varities of numbers or object types # In[21]: ser1 = pd.Series([1,2,3,4],['USA','Germany','USSR','Japan']) ser1 # In[22]: ser2 = pd.Series([1,2,6,4],['USA','Germany','Italy','Japan']) # In[24]: #passing the index of 1 ser1['USA'] # In[27]: ser3 = pd.Series(labs, my_data) ser3 # In[26]: ser3[0] #index labs # In[28]: ser1 # In[29]: ser2 # In[30]: ser1 + ser2 #integer is converted to float # In[ ]: